4Seasons Dataset
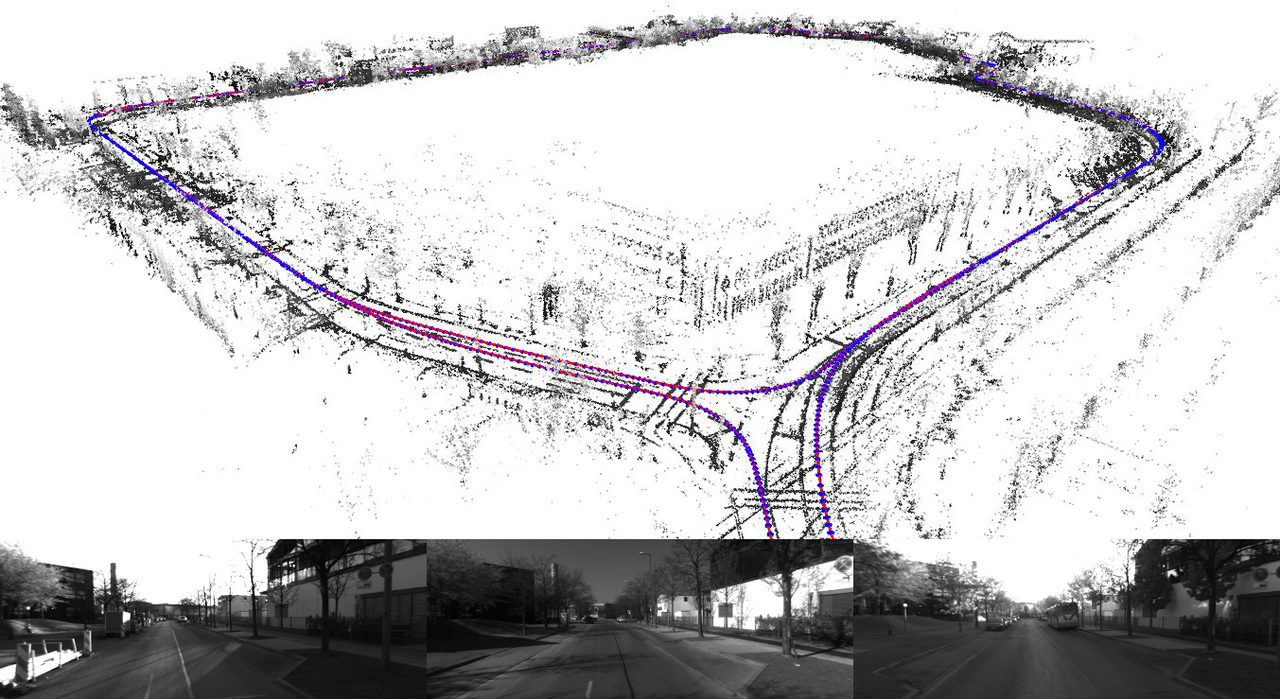
4Seasons: A Cross-Season Dataset for Multi-Weather SLAM in Autonomous Driving
We present a novel dataset covering seasonal and challenging perceptual conditions for autonomous driving. Among others, it enables research on visual odometry, global place recognition, and map-based re-localization tracking. The data was collected in different scenarios and under a wide variety of weather conditions and illuminations, including day and night. This resulted in more than 350 km of recordings in nine different environments ranging from multi-level parking garage over urban (including tunnels) to countryside and highway. We provide globally consistent reference poses with up-to centimeter accuracy obtained from the fusion of direct stereo visual-inertial odometry with RTK-GNSS.
Dataset Tools
To make the work with our dataset as convenient as possible we are providing a collection of python tools in a GitHub repository.
Dataset
You can download the 4Seasons dataset here.
License
All datasets and benchmarks on this page are copyright by Artisense and published under the Creative Commons Attribution-NonCommercial-ShareAlike 4.0 International (CC BY-NC-SA 4.0) License. This means that you must attribute the work in the manner specified by the authors, you may not use this work for commercial purposes and if you alter, transform, or build upon this work, you may distribute the resulting work only under the same license. Per GDPR requirements, to download and use the data you need to register and specify the intended purpose of using the dataset.
Exclusive commercial rights for the 4Seasons dataset are held by Artisense. For commercial licensing of the dataset please contact licensing@artisense.ai.
Sensors
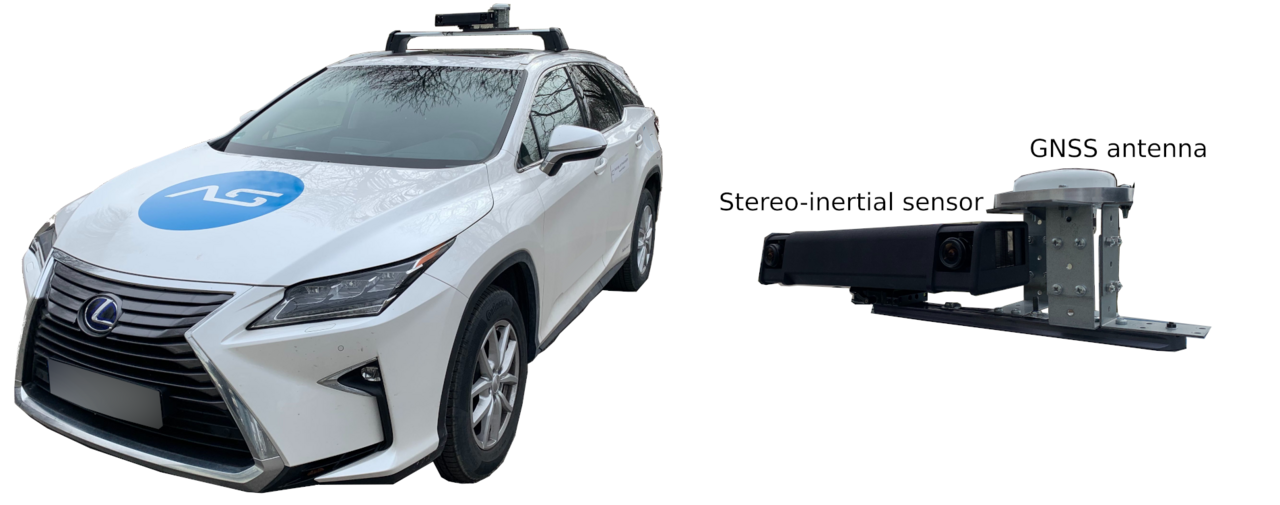
Test vehicle and sensor system (Artisense VINS) used for dataset recording. Our sensor setup consists of two monochrome cameras in a stereo setup and an IMU. The system is coupled with an high-end RTK-GNSS receiver for global positioning. For more details, please refer to the paper.
Citation
If you find our dataset useful, please cite the following paper:
@inproceedings{wenzel2020fourseasons, author = {P. Wenzel and R. Wang and N. Yang and Q. Cheng and Q. Khan and L. von Stumberg and N. Zeller and D. Cremers}, title = {{4Seasons}: A Cross-Season Dataset for Multi-Weather {SLAM} in Autonomous Driving}, booktitle = {Proceedings of the German Conference on Pattern Recognition ({GCPR})}, year = {2020} }
Export as PDF, XML, TEX or BIB
Conference and Workshop Papers
2020
[] 4Seasons: A Cross-Season Dataset for Multi-Weather SLAM in Autonomous Driving , In Proceedings of the German Conference on Pattern Recognition (GCPR), 2020. ([arXiv][video])
Privacy
This dataset is made available for academic use only. However, we take your privacy seriously! If you find yourself or your personal belongings in this dataset and feel unwell about it, please contact us 4seasons-dataset@artisense.ai, and we will immediately remove the respective data from our server.
Related Workshops
We have been organizing workshops at international research conferences (i.e., ECCV, ICCV) to discuss the topic of map-based localization in autonomous driving with experts from industry and academia. In conjunction with the workshop, we are regularly organizing challenges based on the 4Seasons dataset.
Recent workshops: