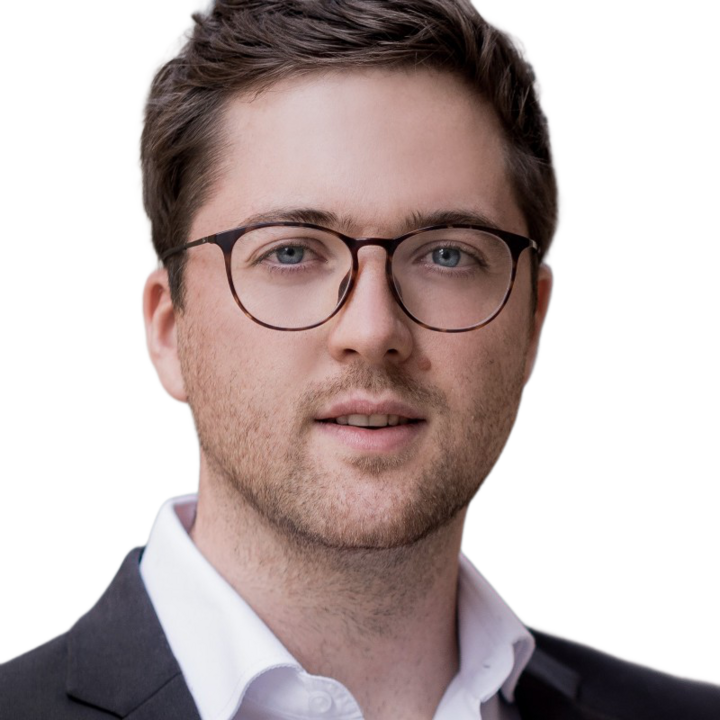
Johannes Michael Meier
PhD StudentTechnical University of MunichSchool of Computation, Information and Technology
Informatics 9
Boltzmannstrasse 3
85748 Garching
Germany
Fax: +49-89-289-17757
Mail: J.Meier@tum.de
Brief Bio
I received my M.Sc. in Computer Science from the University of Tuebingen. I did my master thesis with Bosch Research in Renningen and was supervised by Prof. Dr. Andreas Geiger and Dr. Wieland Brendel of the Max Planck Institute Tuebingen. I am a Ph.D. student in the Computer Vision Group, headed by Prof. Dr. Daniel Cremers at TUM. My research focuses on 3D object detection, tracking, semi-supervised learning and domain adaptation.
Master thesis topics
Are you excited about Computer Vision and Machine Learning? Are you interested in doing high impact work and submitting it to top conferences?
Then apply for one of the Master’s theses below.
I pursue publications in conferences like CVPR, ICCV, and ECCV and solve challenging computer vision problems identified jointly with Deepscenario - a highly innovative start-up in the automotive industry advised by Prof. Daniel Cremers (https://www.deepscenario.com/).
Specific thesis topics:
Master thesis: Domain Adaptation for Monocular 3D object detection in Autonomous Driving
Monocular 3D object detection is a challenging task because it requires models to predict the location, dimensions, and rotation of objects from a single input image. Traditional autonomous driving datasets, such as KITTI, NuScenes, Waymo, and Rope3D, are captured from a car or traffic view perspective. However, this can limit the generalization ability of models trained on these datasets. For example, a model trained on data from a car view may not be able to accurately detect objects from a drone view. In this thesis we want to answer the following research question: How can we generalize monocular 3D object detection models from a set of training perspectives to an unseen perspective during inference?
Master thesis: Generalized Monocular Depth Prediction for Autonomous Driving
Monocular depth prediction is a key challenge for autonomous driving. Current approaches either directly predict depth or use strong assumptions that require the input image to be taken from a specific perspective. This limits the generalization ability of these approaches to different perspectives. This thesis will investigate how to perform accurate depth prediction from any perspective, including car view, traffic view, and drone view.
Student Feedback
I was fortunate to have Johannes Meier as my supervisor during my Master’s thesis. He has been a brilliant mentor, significantly impacting my academic and professional development. His dedication to excellence constantly motivated me to strive for the highest standards in my work. Moreover, his persistent encouragement, deep expertise, and insightful guidance paved the way for me to confidently overcome challenges, ultimately leading us to submit my work to a top-tier computer vision conference. Johannes led by example, demonstrating passion and commitment that inspired me to do the same. What I truly appreciated about Johannes was his genuine interest in my personal and professional growth, even beyond the scope of the thesis. Johannes’ positive mindset and leadership made working with him an incredibly rewarding experience. I genuinely recommend Johannes for any professional role, as his dedication, expertise, and ability to inspire others are truly exceptional.. (Florian Günther, Master Thesis Student in Monocular 3D Detection)
I had the great opportunity to work with Johannes as my Master's thesis supervisor at TUM. His high expectations together with his supportive approach created an environment that encouraged and enabled me to achieve my best work. What truly set Johannes apart was his commitment to my success. Every week, he dedicated a significant amount of time to meet with me, providing valueable feedback and guidance. Johannes was always available when I needed advice and not only helped me professionally but also supported me personally, providing encouragement and motivation during the more difficult phases of my thesis. Johannes’s technical expertise and understanding of complex topics in the field was invaluable in guiding me throughout my research. If you have the opportunity to work with Johannes, I have no doubt that you’ll benefit from his exceptional expertise, mentorship and support. (Jonathan Michel, Master Thesis Student in Monocular 3D Detection)
I had the privilege of being supervised by Johannes during my master's thesis, an experience that significantly shaped my academic and professional development. Johannes consistently demonstrated an exceptional blend of expertise, guidance, and encouragement. His ability to provide insightful feedback and foster a collaborative environment was instrumental in refining my research and achieving notable milestones, including the submission of my work to a top-tier robotics conference. Johannes's dedication to my growth extended beyond the thesis, as he continued to support and mentor me even after the submission, ensuring that I excelled in all aspects of my work. On a personal level, Johannes was a joy to work with. His positive attitude and genuine interest in my success made the entire process not only productive but also enjoyable. His exceptional leadership, coupled with his collaborative spirit, made a profound impact on my academic journey. I highly recommend Johannes for any professional endeavors. His commitment to excellence, passion for research, and dedication to fostering talent make him an invaluable asset to any team or project. (Louis Inchingolo, Master Thesis Student in Domain Adaptation)
Working with Johannes as a mentor during my Master's thesis was a great experience. Johannes proved to be an exceptional supervisor who is focused, dedicated and highly supportive. I benefited greatly from Johannes' extensive knowledge and insightful feedback on several occasions, which meaningfully impacted the quality and depth of my work. I would recommend Johannes for any future mentorship or professional role where his expertise and supportive approach would be an asset. (Luca Scalerandi, Master Thesis Student in 3D Tracking)
Working with Johannes was an invaluable opportunity that significantly enriched my experience. He consistently provided constructive feedback and technical support, enabling me to enhance my understanding and improve the quality of my work. Johannes is approachable, responsive, and always open to engaging in meaningful discussions. His supervision was both supportive and motivating, often involving hands-on collaboration to solve complex problems. His guidance not only elevated the quality of my research but also deepened my knowledge of the field. I wholeheartedly recommend Johannes as a supervisor. (Melih Görgülü, Master Thesis Student in Monocular 3D Detection)
Publications
Export as PDF, XML, TEX or BIB
Conference and Workshop Papers
2025
[] Shape Your Ground: Refining Road Surfaces Beyond Planar Representations , In 2025 IEEE Intelligent Vehicles Symposium, 2025. ([project page])
[] Highly Accurate and Diverse Traffic Data: The DeepScenario Open 3D Dataset , In 2025 IEEE Intelligent Vehicles Symposium, 2025. ([project page])
[] MonoCT: Overcoming Monocular 3D Detection Domain Shift with Consistent Teacher Models , In International Conference on Robotics and Automation (ICRA), 2025.
Oral Presentation
2024
[] CARLA Drone: Monocular 3D Object Detection from a Different Perspective , In 46th German Conference on Pattern Recognition (GCPR), 2024. ([project page])
Oral Presentation
2023
[] NIFF: Alleviating Forgetting in Generalized Few-Shot Object Detection via Neural Instance Feature Forging , In IEEE Conference on Computer Vision and Pattern Recognition (CVPR), 2023.
2021
[] A Robust Unsupervised Ensemble of Feature-Based Explanations using Restricted Boltzmann Machines , In Neural Information Processing Systems Conference - NeurIPS 2021: eXplainable AI approaches for debugging and diagnosis workshop, 2021.