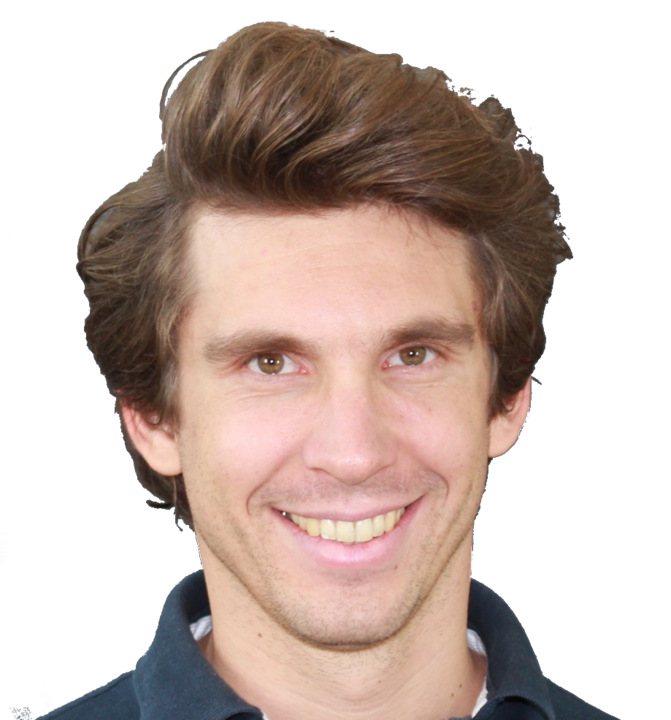
Christian Tomani
PhD StudentTechnical University of MunichSchool of Computation, Information and Technology
Informatics 9
Boltzmannstrasse 3
85748 Garching
Germany
Tel: +49-89-289-17779
Fax: +49-89-289-17757
Office: 02.09.037
Mail: christian.tomani@in.tum.de
Brief Bio
Find me on Linkedin and Google Scholar.
I am a PhD student at the Technical University of Munich at the Chair of Prof. Daniel Cremers. I received my Master's degree from TUM and my Bachelor's degree from Technical University Graz and studied as well as conducted research at University of Oxford, University of California Berkeley and University of Agder. I worked at Google, Meta and Siemens as a research intern.
Research Internships in Industry:
Meta (New York)
Paper: Uncertainty-Based Abstention in LLMs Improves Safety and Reduces Hallucinations (C Tomani, Kamalika C, Ivan E, Daniel C, Mark I), In arXiv preprint, 2024.
Google (San Francisco Bay Area)
Paper: Quality Control at Your Fingertips: Quality-Aware Translation Models (C Tomani, D Vilar and M Freitag and C Cherry and S Naskar and M Finkelstein and D Cremers), In arXiv preprint, 2023.
Siemens (Munich)
Paper: Towards Trustworthy Predictions from Deep Neural Networks with Fast Adversarial Calibration (C Tomani and F Buettner), AAAI, 2021.
Research Visits in Academia:
University of Oxford
Machine Learning Group - Department of Engineering Science
University of California Berkeley
Artificial Intelligence Research Lab (BAIR) - Redwood Center for Theoretical Neuroscience
My Work
I am interested in developing reliable, robust, and reasoning-based large language models (LLMs) and multimodal models. Moreover, I am fascinated by enhancing the reasoning ability, safety and uncertainty awareness of generative models through pre-training and post-training (via fine-tuning and alignment to human preferences) to develop grounded world models and improve factuality and trustworthiness.
My work covers a large spectrum of Machine Learning and Deep Learning topics. Projects of mine include reliable, reasoning-based, safe and uncertainty aware models for in domain, domain shift and out of domain (OOD) scenarios; Natural Language Processing (NLP) and Large Language Models (LLMs); investigating reasoning capabilities and developing reliable LLMs; Computer Vision (CV); Time Series Data Analysis with supervised and self-supervised learning algorithms; Recurrent Neural Networks (RNNs) and Transformer architectures; attribution maps; designing learning algorithms for generalization; etc.
Publications
- Beyond In-Domain Scenarios: Robust Density-Aware Calibration, ICML 2023.
- Parameterized Temperature Scaling for Boosting the Expressive Power in Post-Hoc Uncertainty Calibration, ECCV 2022.
- Post-hoc Uncertainty Calibration for Domain Drift Scenarios, CVPR 2021, Oral Presentation.
Export as PDF, XML, TEX or BIB
2024
Preprints
[] Uncertainty-Based Abstention in LLMs Improves Safety and Reduces Hallucinations , In arXiv preprint, 2024.
2023
Preprints
[] Quality Control at Your Fingertips: Quality-Aware Translation Models , In arXiv preprint, 2023.
Conference and Workshop Papers
[] Beyond In-Domain Scenarios: Robust Density-Aware Calibration , In Proceedings of the 40th International Conference on Machine Learning (ICML), 2023.
2022
Preprints
[] Challenger: Training with Attribution Maps , In arXiv preprint, 2022.
Conference and Workshop Papers
[] What Makes Graph Neural Networks Miscalibrated? , In NeurIPS, 2022. ([code])
[] Parameterized Temperature Scaling for Boosting the Expressive Power in Post-Hoc Uncertainty Calibration , In European Conference on Computer Vision (ECCV), 2022.
2021
Conference and Workshop Papers
[] Post-hoc Uncertainty Calibration for Domain Drift Scenarios , In IEEE Conference on Computer Vision and Pattern Recognition (CVPR), 2021.
Oral Presentation [] Towards Trustworthy Predictions from Deep Neural Networks with Fast Adversarial Calibration , In InThirty-FifthAAAIConferenceonArtificialIntelligence(AAAI-2021), 2021.