Monocular Visual Odometry Dataset
We present a dataset for evaluating the tracking accuracy of monocular Visual Odometry (VO) and SLAM methods. It contains 50 real-world sequences comprising over 100 minutes of video, recorded across different environments – ranging from narrow indoor corridors to wide outdoor scenes.
All sequences contain mostly exploring camera motion, starting and ending at the same position: this allows to evaluate tracking accuracy via the accumulated drift from start to end, without requiring ground-truth for the full sequence.
In contrast to existing datasets, all sequences are photometrically calibrated: We provide the exposure times for each frame as reported by the sensor, the camera response function and the lens attenuation factors (vignetting). Further, we propose a simple approach to non-parametric vignette and camera response calibration, which require minimal set-up and are easy to reproduce.
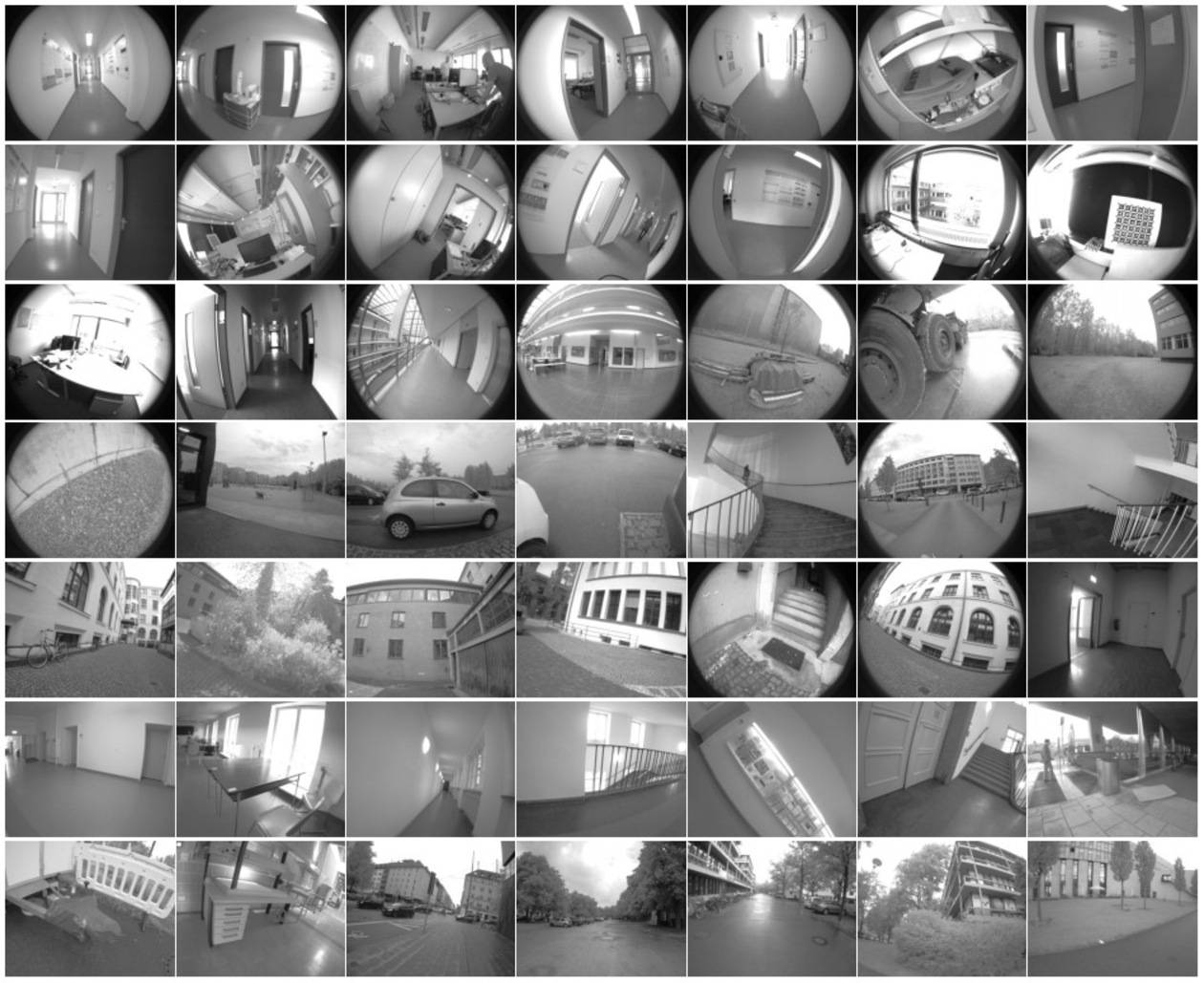
Dataset Download
- Full dataset with all 50 sequences zip (43GB)
- Code for reading and undistorting the dataset sequences; performing photometric calibration with proposed approach https://github.com/tum-vision/mono_dataset_code
- More notes on the intrinsic calibration format: https://github.com/JakobEngel/dso#31-dataset-format
- Supplementary material with ORB-SLAM and DSO results zip (2.7GB) and Matlab scripts for running evaluation zip (30MB) (14.10.2016.: We have updated the supplementary material with the fixed real-time results for ORB-SLAM, corresponding to the revised version of the papers.)
- Individual sequences:
Sequence name | Number of frames | Duration | FPS | Min exposure | Max exposure | Download | Preview Video | Preview Video Rectified |
sequence_01 | 4757 | 95.13s | 50.02 | 1.66ms | 19.98ms | zip (1.30GB) | play (5x) | play (5x) |
sequence_02 | 3500 | 69.98s | 50.01 | 2.64ms | 19.98ms | zip (0.88GB) | play (5x) | play (5x) |
sequence_03 | 5427 | 108.55s | 50.00 | 0.24ms | 13.93ms | zip (1.51GB) | play (5x) | play (5x) |
sequence_04 | 6921 | 138.47s | 50.00 | 1.01ms | 19.98ms | zip (1.83GB) | play (5x) | play (5x) |
sequence_05 | 6300 | 125.97s | 50.01 | 0.11ms | 19.98ms | zip (2.01GB) | play (5x) | play (5x) |
sequence_06 | 4500 | 90.04s | 50.01 | 2.81ms | 19.98ms | zip (1.21GB) | play (5x) | play (5x) |
sequence_07 | 3556 | 71.10s | 50.01 | 0.87ms | 7.45ms | zip (0.94GB) | play (5x) | play (5x) |
sequence_08 | 4300 | 86.08s | 50.01 | 1.09ms | 19.98ms | zip (1.01GB) | play (5x) | play (5x) |
sequence_09 | 2300 | 46.00s | 50.04 | 5.03ms | 15.03ms | zip (0.38GB) | play (5x) | play (5x) |
sequence_10 | 2100 | 41.98s | 50.01 | 4.76ms | 17.09ms | zip (0.36GB) | play (5x) | play (5x) |
sequence_11 | 1500 | 59.95s | 25.00 | 0.58ms | 17.47ms | zip (0.25GB) | play (5x) | play (5x) |
sequence_12 | 2250 | 89.99s | 24.99 | 0.14ms | 16.28ms | zip (0.38GB) | play (5x) | play (5x) |
sequence_13 | 1800 | 71.98s | 24.99 | 0.47ms | 32.13ms | zip (0.28GB) | play (5x) | play (5x) |
sequence_14 | 1550 | 61.93s | 25.02 | 3.86ms | 39.94ms | zip (0.23GB) | play (5x) | play (5x) |
sequence_15 | 2700 | 107.91s | 25.01 | 0.32ms | 32.27ms | zip (0.50GB) | play (5x) | play (5x) |
sequence_16 | 1850 | 73.93s | 25.01 | 4.95ms | 23.50ms | zip (0.30GB) | play (5x) | play (5x) |
sequence_17 | 4980 | 124.39s | 40.03 | 0.34ms | 2.37ms | zip (0.99GB) | play (5x) | play (5x) |
sequence_18 | 6200 | 154.94s | 40.01 | 0.68ms | 4.30ms | zip (1.12GB) | play (5x) | play (5x) |
sequence_19 | 8380 | 209.43s | 40.00 | 0.09ms | 1.00ms | zip (1.65GB) | play (5x) | play (5x) |
sequence_20 | 5380 | 134.45s | 40.00 | 0.11ms | 12.96ms | zip (1.10GB) | play (5x) | play (5x) |
sequence_21 | 5470 | 273.68s | 20.00 | 0.05ms | 1.89ms | zip (1.47GB) | play (5x) | play (5x) |
sequence_22 | 6340 | 316.98s | 20.00 | 0.06ms | 0.28ms | zip (1.79GB) | play (5x) | play (5x) |
sequence_23 | 3740 | 124.64s | 29.99 | 0.42ms | 3.17ms | zip (0.89GB) | play (5x) | play (5x) |
sequence_24 | 3500 | 116.64s | 29.99 | 0.45ms | 3.83ms | zip (0.77GB) | play (5x) | play (5x) |
sequence_25 | 4090 | 136.31s | 29.99 | 0.41ms | 3.87ms | zip (0.96GB) | play (5x) | play (5x) |
sequence_26 | 2760 | 91.95s | 30.01 | 6.35ms | 33.31ms | zip (0.36GB) | play (5x) | play (5x) |
sequence_27 | 3480 | 115.98s | 30.02 | 0.03ms | 0.16ms | zip (0.69GB) | play (5x) | play (5x) |
sequence_28 | 5550 | 185.31s | 30.01 | 0.37ms | 33.29ms | zip (0.70GB) | play (5x) | play (5x) |
sequence_29 | 8400 | 280.03s | 30.02 | 0.01ms | 0.69ms | zip (2.30GB) | play (5x) | play (5x) |
sequence_30 | 1800 | 85.30s | 21.09 | 0.01ms | 0.21ms | zip (0.45GB) | play (5x) | play (5x) |
sequence_31 | 3240 | 153.59s | 21.09 | 0.01ms | 0.26ms | zip (0.85GB) | play (5x) | play (5x) |
sequence_32 | 2700 | 127.99s | 21.09 | 0.01ms | 0.26ms | zip (0.72GB) | play (5x) | play (5x) |
sequence_33 | 2760 | 91.92s | 30.01 | 0.02ms | 0.43ms | zip (0.68GB) | play (5x) | play (5x) |
sequence_34 | 4290 | 203.38s | 21.09 | 0.02ms | 0.76ms | zip (1.12GB) | play (5x) | play (5x) |
sequence_35 | 2550 | 85.06s | 30.00 | 5.47ms | 33.31ms | zip (0.36GB) | play (5x) | play (5x) |
sequence_36 | 2350 | 78.29s | 30.00 | 6.01ms | 33.31ms | zip (0.32GB) | play (5x) | play (5x) |
sequence_37 | 2970 | 98.96s | 30.00 | 3.63ms | 33.31ms | zip (0.38GB) | play (5x) | play (5x) |
sequence_38 | 3330 | 133.17s | 25.00 | 1.19ms | 10.95ms | zip (0.37GB) | play (5x) | play (5x) |
sequence_39 | 3540 | 141.65s | 24.99 | 1.52ms | 14.56ms | zip (0.38GB) | play (5x) | play (5x) |
sequence_40 | 4350 | 174.42s | 25.00 | 1.22ms | 13.05ms | zip (0.44GB) | play (5x) | play (5x) |
sequence_41 | 3100 | 123.98s | 24.99 | 5.34ms | 25.52ms | zip (0.42GB) | play (5x) | play (5x) |
sequence_42 | 4830 | 224.49s | 21.53 | 0.02ms | 10.59ms | zip (1.14GB) | play (5x) | play (5x) |
sequence_43 | 2160 | 100.28s | 21.53 | 0.05ms | 1.12ms | zip (0.66GB) | play (5x) | play (5x) |
sequence_44 | 2100 | 97.50s | 21.53 | 0.03ms | 15.00ms | zip (0.45GB) | play (5x) | play (5x) |
sequence_45 | 3000 | 99.99s | 29.99 | 0.14ms | 0.66ms | zip (0.93GB) | play (5x) | play (5x) |
sequence_46 | 4110 | 137.07s | 29.99 | 0.08ms | 2.08ms | zip (1.02GB) | play (5x) | play (5x) |
sequence_47 | 3260 | 129.84s | 25.13 | 0.35ms | 4.26ms | zip (0.99GB) | play (5x) | play (5x) |
sequence_48 | 3250 | 129.41s | 25.13 | 0.36ms | 4.74ms | zip (0.96GB) | play (5x) | play (5x) |
sequence_49 | 3255 | 129.48s | 25.13 | 0.21ms | 1.78ms | zip (0.82GB) | play (5x) | play (5x) |
sequence_50 | 4050 | 161.12s | 25.13 | 0.27ms | 1.65ms | zip (1.12GB) | play (5x) | play (5x) |
Calibration sequences
- All calibration sequences zip (13GB)
- Individual sequences:
Sequence name | Download | Preview Video |
narrowGamma_scene1 | zip (2.02GB) | play (5x) |
narrowGamma_scene2 | zip (1.20GB) | play (5x) |
narrowGamma_sweep1 | zip (0.37GB) | play (5x) |
narrowGamma_sweep2 | zip (0.76GB) | play (5x) |
narrowGamma_sweep3 | zip (0.29GB) | play (5x) |
narrowGamma_vignette | zip (0.35GB) | play (5x) |
narrow_checkerboard1 | zip (0.27GB) | play (5x) |
narrow_checkerboard2 | zip (0.05GB) | |
narrow_sweep1 | zip (0.51GB) | play (5x) |
narrow_sweep2 | zip (0.40GB) | play (5x) |
narrow_sweep3 | zip (0.20GB) | play (5x) |
narrow_vignette | zip (0.32GB) | play (5x) |
narrow_whitePaper | zip (0.04GB) | play (5x) |
wideGamma_scene1 | zip (1.56GB) | play (5x) |
wideGamma_sweep1 | zip (0.53GB) | play (5x) |
wideGamma_sweep2 | zip (0.70GB) | play (5x) |
wideGamma_vignette | zip (0.41GB) | play (5x) |
wideGamma_vignette2 | zip (0.42GB) | play (5x) |
wide_checkerboard1 | zip (0.24GB) | play (5x) |
wide_checkerboard2 | zip (0.04GB) | |
wide_sweep1 | zip (0.46GB) | play (5x) |
wide_sweep2 | zip (0.59GB) | play (5x) |
wide_vignette | zip (0.55GB) | play (5x) |
wide_vignette2 | zip (0.27GB) | play (5x) |
wide_whitePaper | zip (0.06GB) | play (5x) |
License
Unless stated otherwise, all data in the Monocular Visual Odometry Dataset is licensed under a Creative Commons 4.0 Attribution License (CC BY 4.0) and the accompanying source code is licensed under a BSD-2-Clause License.
Publications
Export as PDF, XML, TEX or BIB
Journal Articles
2022
[] DM-VIO: Delayed Marginalization Visual-Inertial Odometry , In IEEE Robotics and Automation Letters (RA-L) & International Conference on Robotics and Automation (ICRA), volume 7, 2022. ([arXiv][video][project page][supplementary][code])
2018
[] Omnidirectional DSO: Direct Sparse Odometry with Fisheye Cameras , In IEEE Robotics and Automation Letters & Int. Conference on Intelligent Robots and Systems (IROS), IEEE, 2018. ([arxiv])
[] Online Photometric Calibration of Auto Exposure Video for Realtime Visual Odometry and SLAM , In IEEE Robotics and Automation Letters (RA-L), volume 3, 2018. (This paper was also selected by ICRA'18 for presentation at the conference.[arxiv][video][code][project])
ICRA'18 Best Vision Paper Award - Finalist [] Direct Sparse Odometry , In IEEE Transactions on Pattern Analysis and Machine Intelligence, 2018.
Conference and Workshop Papers
2021
[] Tight Integration of Feature-based Relocalization in Monocular Direct Visual Odometry , In Proc. of the IEEE International Conference on Robotics and Automation (ICRA), 2021. ([project page])
2020
[] D3VO: Deep Depth, Deep Pose and Deep Uncertainty for Monocular Visual Odometry , In IEEE Conference on Computer Vision and Pattern Recognition (CVPR), 2020.
Oral Presentation
2019
[] Rolling-Shutter Modelling for Visual-Inertial Odometry , In International Conference on Intelligent Robots and Systems (IROS), 2019. ([arxiv])
2018
[] Direct Sparse Odometry With Rolling Shutter , In European Conference on Computer Vision (ECCV), 2018. ([supplementary][arxiv])
Oral Presentation [] Deep Virtual Stereo Odometry: Leveraging Deep Depth Prediction for Monocular Direct Sparse Odometry , In European Conference on Computer Vision (ECCV), 2018. ([arxiv],[supplementary],[project])
Oral Presentation [] LDSO: Direct Sparse Odometry with Loop Closure , In International Conference on Intelligent Robots and Systems (IROS), 2018. ([arxiv][video][code][project])
[] Direct Sparse Visual-Inertial Odometry using Dynamic Marginalization , In International Conference on Robotics and Automation (ICRA), 2018. ([supplementary][video][arxiv])
2017
[] Stereo DSO: Large-Scale Direct Sparse Visual Odometry with Stereo Cameras , In International Conference on Computer Vision (ICCV), 2017. ([supplementary][video][arxiv][project])
2016
[] Direct Sparse Odometry , In arXiv:1607.02565, 2016.
[] A Photometrically Calibrated Benchmark For Monocular Visual Odometry , In arXiv:1607.02555, 2016.