DH3D: Deep Hierarchical 3D Descriptors for Robust Large-Scale 6DoF Relocalization
Abstract
For relocalization in large-scale point clouds, we propose the first approach that unifies global place recognition and local 6DoF pose refinement. To this end, we design a Siamese network that jointly learns 3D local feature detection and description directly from raw 3D points. It integrates FlexConv and Squeeze-and-Excitation (SE) to assure that the learned local descriptor captures multi-level geometric information and channel-wise relations. For detecting 3D keypoints we predict the discriminativeness of the local descriptors in an unsupervised manner. We generate the global descriptor by directly aggregating the learned local descriptors with an effective attention mechanism. In this way, local and global 3D descriptors are inferred in one single forward pass. Experiments on various benchmarks demonstrate that our method achieves competitive results for both global point cloud retrieval and for local point cloud registration in comparison to state-of-the-art approaches. To validate the generalizability and robustness of our 3D keypoints, we demonstrate that our method also performs favorably without fine-tuning on registration of point clouds that were generated by a visual SLAM system.
Update
- [11.11.2020] For the ease of comparison, we upload the numbers used to draw the plots in the paper (download).
ECCV Spotlight Presentation
The video is with audio.
Citation
If you find our work useful in your research, please consider citing:
@inproceedings{du2020dh3d, title={DH3D: Deep Hierarchical 3D Descriptors for Robust Large-Scale 6DoF Relocalization}, author={Du, Juan and Wang, Rui and Cremers, Daniel}, booktitle={European Conference on Computer Vision (ECCV)}, year={2020} }
Code
Code and the pre-trained models can be accessed from the GitHub page.
Datasets
Our model is mainly trained and tested on the LiDAR point clouds from the Oxford RobotCar dataset. To test the generalization capability, two extra datasets are used, namely ETH (LiDAR point clouds from two sequences, gazebo_winter and wood_autumn) and Oxford RobotCar Stereo DSO (point clouds generated by running Stereo DSO). As our method can be used for global place recognition (retrieval) and local pose refinement (regression), the corresponding datasets are denoted by "global" and "local" respectively. For more details on how the datasets are generated, please refer to the beginning of Section 4 in the main paper and Section 4.2 and 4.4 in the supplementary material. For examples on how to train or test our model on these datasets, please refer to the GitHub page.
Dataset | Point Clouds | Ground Truth |
Training | ||
Oxford RobotCar (local and global) | zip (3.1GB) | local_gt.pickle, global_gt.pickle |
Testing | ||
Oxford RobotCar (local) | zip (294MB) | gt.txt |
Oxford RobotCar (global) | zip (740MB) | query_gt.pickle, reference_gt.pickle |
Generalization Testing | ||
ETH gazebo_winter (local) | zip (11MB) | gt.txt | ETH wood_autumn (local) | zip (16MB) | gt.txt |
Oxford RobotCar Stereo DSO (local) | zip (44MB) | gt.txt |
Qualitative Results
- Resuts on LiDAR points of Oxford RobotCar
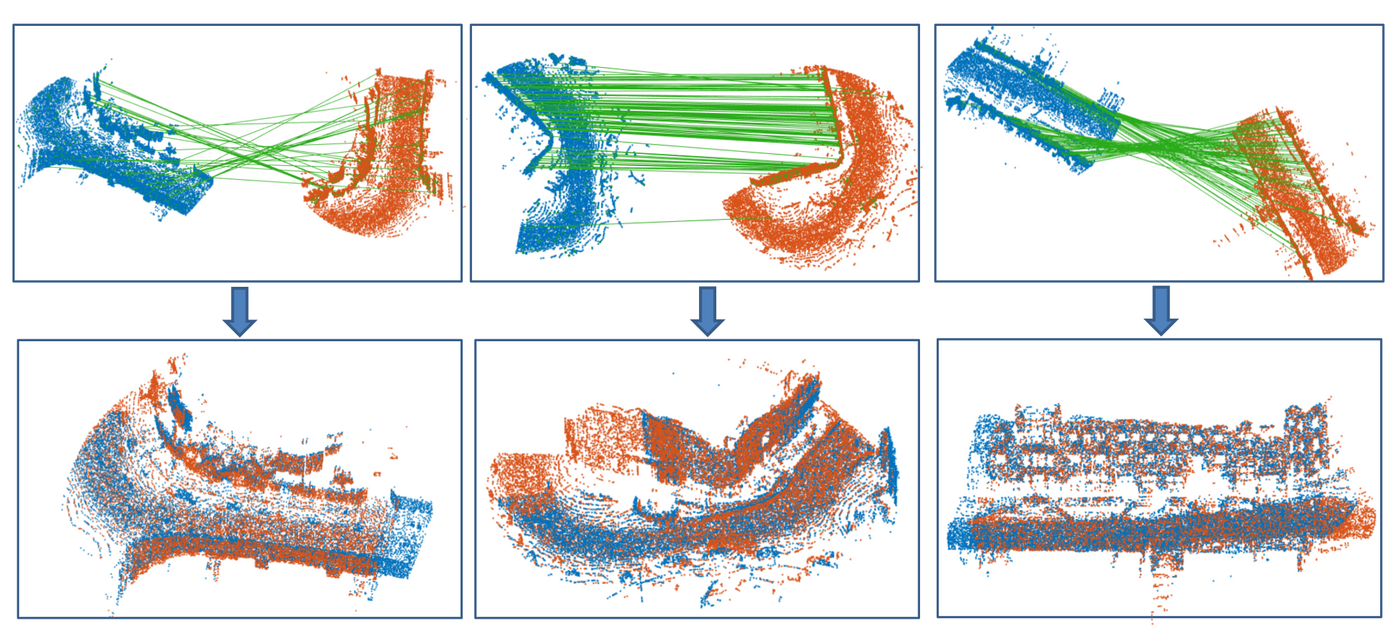

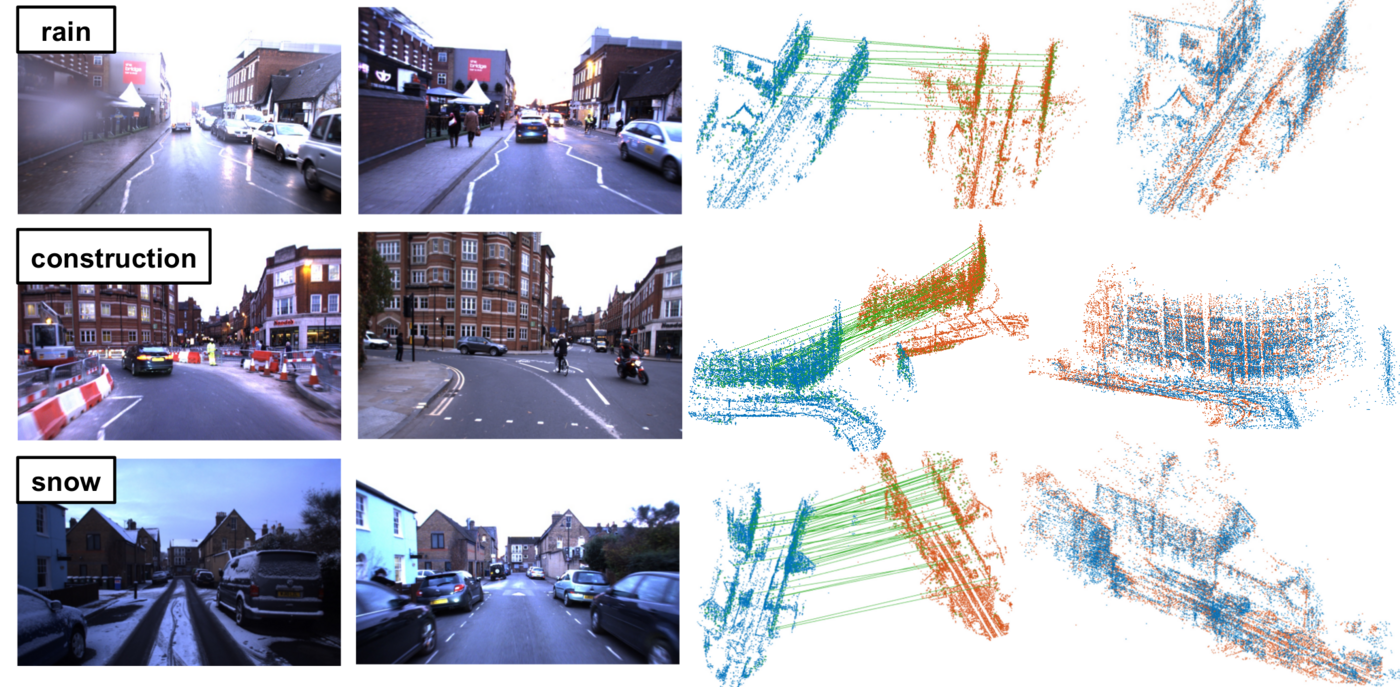
Other Materials
Publications
Export as PDF, XML, TEX or BIB
Conference and Workshop Papers
2020
[] DH3D: Deep Hierarchical 3D Descriptors for Robust Large-Scale 6DoF Relocalization , In European Conference on Computer Vision (ECCV), 2020. ([project page][code][supplementary][arxiv])
Spotlight Presentation
2017
[] Stereo DSO: Large-Scale Direct Sparse Visual Odometry with Stereo Cameras , In International Conference on Computer Vision (ICCV), 2017. ([supplementary][video][arxiv][project])